Table of contents
Conclusions
- This small study adds to a growing body of research that suggests creating plain language summaries is possible for genAI.
- With minimal coaching, ChatGPT can generate PLS abstracts that are at least as understandable as human-written PLS abstracts.
- However, it is essential that humans are involved in optimizing prompts and checking quality of outputs to ensure accuracy of technical points, that all important findings are covered and that there is no overinterpretation or simplification.
- Who the right audience is to judge the understandability of a plain language summary should be considered when writing them as this study has shown those with PLS writing or scientific services backgrounds may have somewhat different opinions to those without.
Introduction
- We present an initial assessment of the capabilities of ChatGPT4.0, as well as the prompting strategy, to develop an abstract PLS of a published clinical study.
Methods
- The assessment was conducted in June and July 2023.
-
We selected the most recently published manuscript PLS available online on the Future Medicine website (https://www.futuremedicine.com/plainlanguagesummaries) and identified the primary manuscript publication from the same study.1,2
- Using the abstract from the primary manuscript,2 ChatGPT4.0 was prompted to generate a PLS in the same style of two example PLS (first ChatGPT option).
- Feedback was given to ChatGPT on this first attempt with prompts for improvement.
- Over 5 minutes, three iterations were generated to give a hybrid AI-human ChatGPT abstract (human-assisted ChatGPT option).
- An experienced medical writer checked the ChatGPT-generated abstracts for accuracy.
-
A survey was sent by email (see survey questions) to 341 employees of a medical communications agency to assess and compare understandability of the two ChatGPT options with the humanwritten abstract of the published manuscript PLS.1
- Respondents were asked, on a scale of 1 to 4 (1=poor; 4=high), how understandable they thought each of the abstracts in turn would be to someone with a reading age of 16 years.
- They were also asked to rank the two ChatGPT abstracts and human-written abstract in the order they believed most appropriate for a PLS.
- Respondents were blinded to abstract source and were not told that any of the abstracts had been generated by ChatGPT.
- We also used the Flesch-Kincaid calculator as an objective measure of readability score of each abstract (https://charactercalculator.com/flesch-reading-ease/).
- The survey questions and text of the two ChatGPT abstracts can be accessed via links in the supplementary materials section below.
Results
- In total, there were 39 respondents in the survey (11% response rate), from various job roles and experience levels; 14 (36%) had previous PLS writing experience.
-
An experienced medical writer checked the abstracts generated by ChatGPT against
the primary manuscript2 for accuracy, completeness and potential overinterpretation
(findings shown in Table 1).
- ChatGPT had done a credible job, but the quality check emphasized the need for human oversight to ensure accuracy, and no omissions or overinterpretation.
First ChatGPT abstract | Human-assisted ChatGPT abstract |
|
|
ALK, anaplastic lymphoma kinase; PFS, progression-free survival
- The Flesch-Kincaid Reading Ease score was 48.9 for the first ChatGPT option (college level; difficult to read), 51.8 for the human-assisted ChatGPT option (10th–12th grade; fairly difficult to read) and 54.2 for the human-written abstract (10th–12th grade; fairly difficult to read) (Figure 1A).
- The human-assisted ChatGPT abstract achieved a similar score for understandability by a 16-year-old in the survey to the human-written abstract from the manuscript PLS (Figure 1B).
Figure 1.
Flesch-Kincaid Reading Ease score (A) and Respondent-Assessed Understandability score† (B)
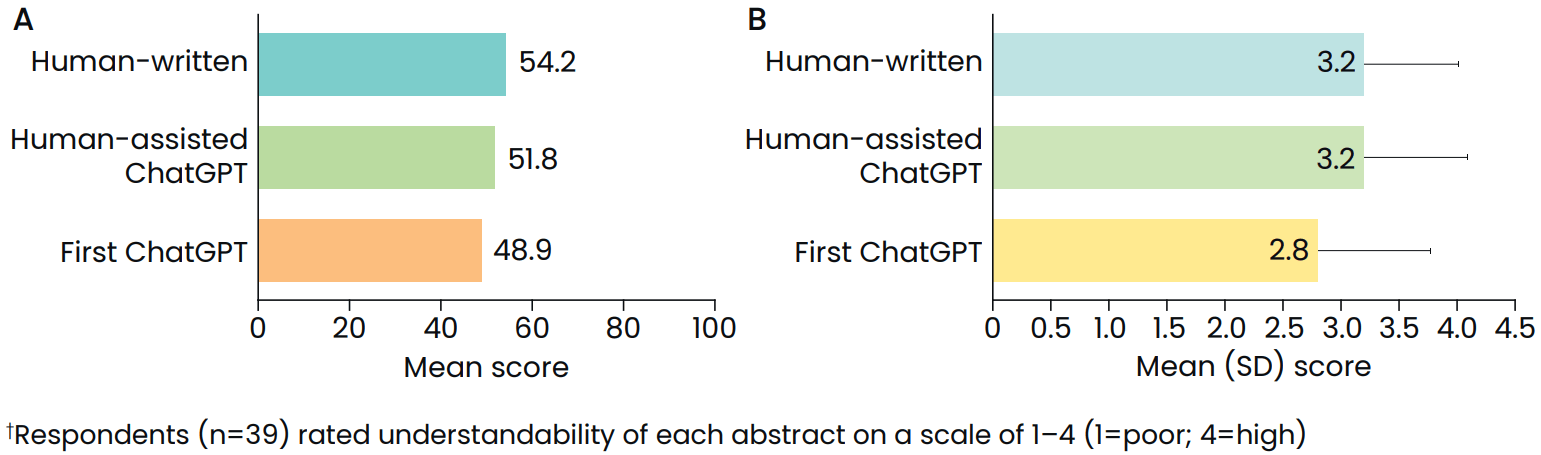
- Figure 2 shows the respondent ratings for understandability according to previous PLS experience and job role.
Figure 2.
Impact of previous experience in PLS and job role on respondent ratings
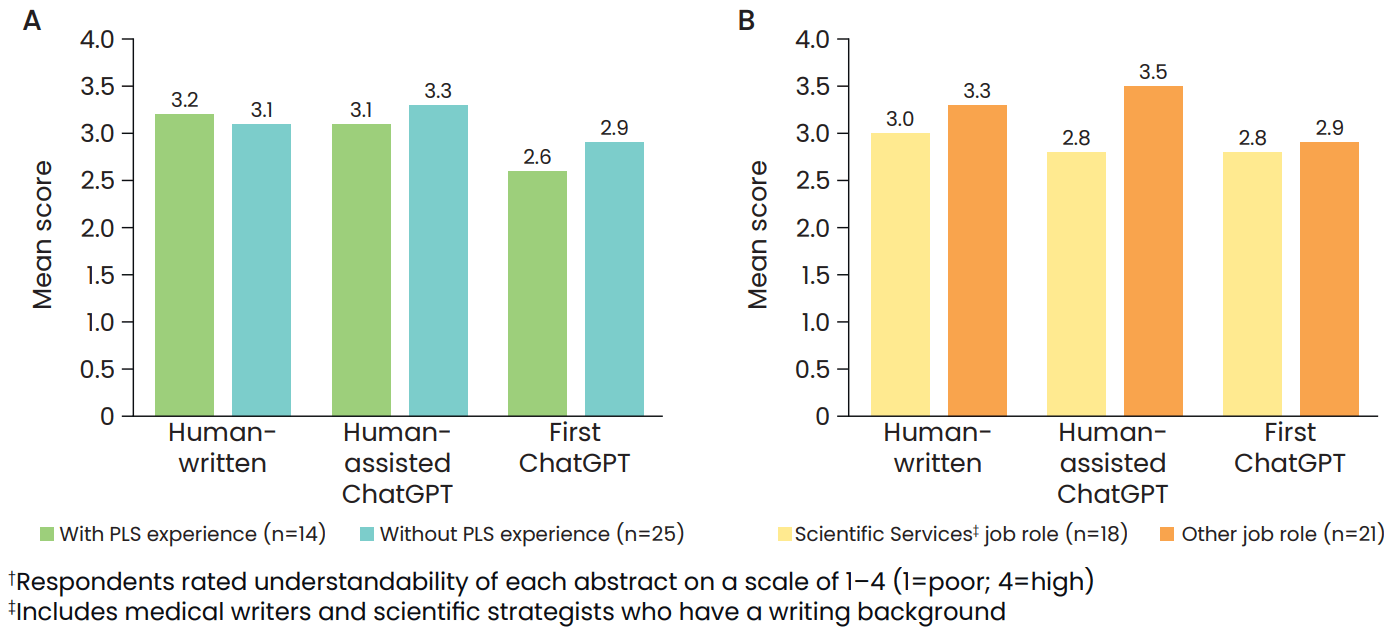
- Overall, 17 respondents (44%) placed the human-written abstract in first place for perceived appropriateness for a PLS, 14 (36%) placed the human-assisted ChatGPT option in first place and eight (21%) placed the first ChatGPT option in first place (Figure 3).
Figure 3.
Perceived appropriateness for a PLS
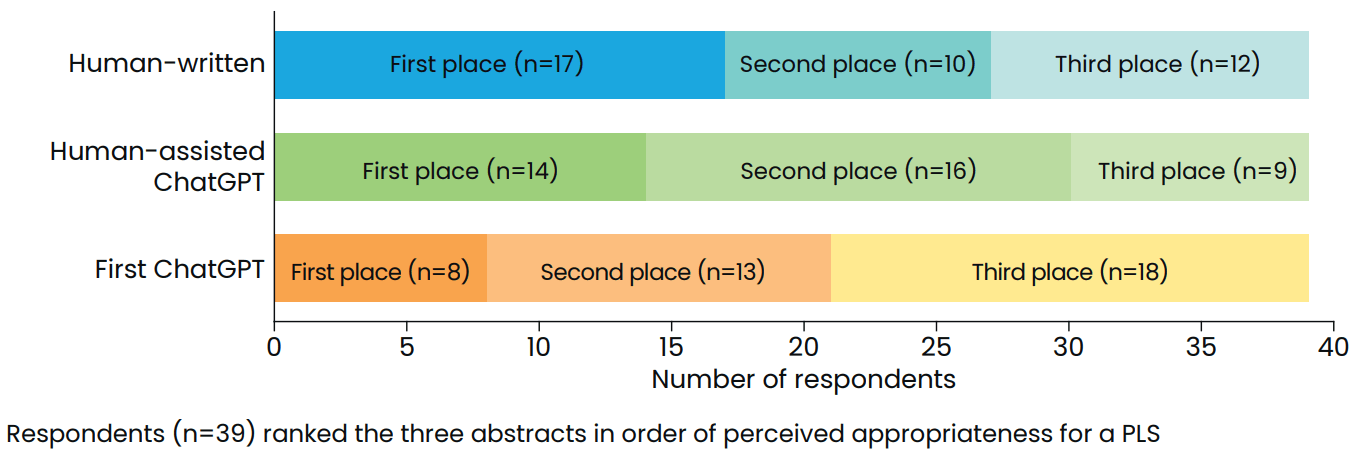
Figure 5.
Attributes that led respondents to prefer their top choice
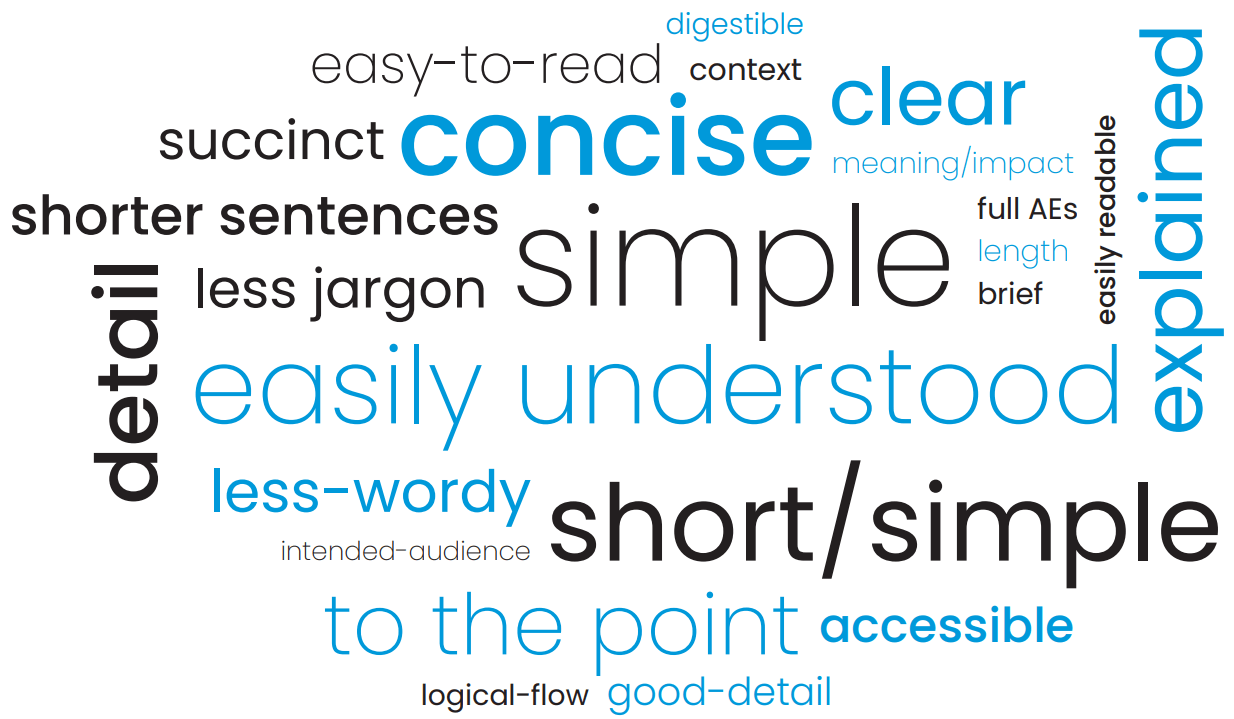
Limitations and future research
- This study was designed to assess the potential for generative AI to develop understandable PLS, as well as prompting strategy, and was not a formal assessment of the accuracy of the outputs.
-
Journals may recommend different styles and reading ages for PLS, and readers may also have preferences for certain styles.3
- The ability of generative AI to tailor responses to different audiences and styles may be particularly beneficial in this area and is an area of future research.
- Future research should also include assessment of patient preferences of PLS styles
- Additionally, further evaluation and definition of the criteria for quality checking of AI-generated content is needed.
References
- Solomon BJ, et al. Future Oncol. 2023;19:961–973.
- Solomon BJ, et al. Lancet Respir Med. 2023;11:354–366.
- Silvagnoli LM. et al. J Med Internet Res. 2022;24:e22122.
Acknowledgements
We would like to thank the Prime Production and Editor teams for their support with developing this poster.
Disclosures
Brian Norman and Valerie Moss are employees of Prime, London, UK. Jenny Ghith is an employee of Pfizer Inc. The research and interpretation provided here represent the views of the authors and not necessarily those of their employers.